Tiago Marques, PhD
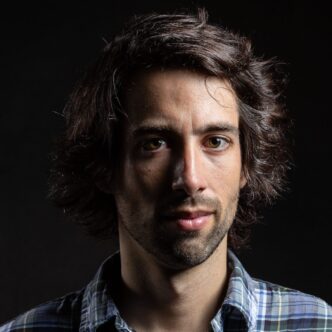
Building an Understanding of Visual Processing in the Brain Via New Neural Network Models
Summary
Potential treatments for blindness, such as neuroprosthetics, require an understanding on how cortical areas in the brain represent visual information. For over half a century, neuroscientists have been studying the primate cortical ventral stream and its role in visual object recognition; that is, the ability to quickly recognize objects in visual scenes. Despite significant progress, we still do not fully understand the sequence of computations that enable this complex visual behavior. Recently, deep artificial neural networks (ANNs) brought a leap in performance in visual object recognition tasks. In addition, ANNs have unprecedented accuracy in predicting the patterns of neuronal responses in the primate visual cortex. Despite being the most accurate model class to describe primate visual processing, the extent of their ability to explain a wide range of neuronal and behavior effects remains limited. This project takes advantage of ANNs to build a more detailed understanding of visual processing in the brain by pursuing two complementary aims: (1) a comprehensive meta-analysis of state-of-the-art models of primate vision, followed by (2) the development of more accurate and brain-mappable biologically-inspired neural networks. By making comparisons with primate neural data, it was first observed that ANNs that better match the primate primary visual cortex (V1) are also more robust to image perturbations. Inspired by this observation, the VOneNet, a new class of hybrid ANN vision models, was developed. This novel network architecture contains a fixed front-end that simulates visual processing in V1, followed by a more traditional back-end adapted from current ANN models. VOneNets are not only substantially more robust than standard ANN models to a wide range of image perturbations, but they also better predict primate neuronal responses in visual cortical areas. These results demonstrate that more precisely mimicking the primate visual system leads to new gains in challenging computer vision applications. The development of even more biologically-matched models will point the way to a deeper understanding of the mechanisms underlying visual perception. This will have important implications, laying the foundations for the development of future therapeutics for vision and enabling more robust computer-assisted medical imaging diagnostics.
Thanks to the PhRMA Foundation Postdoctoral Fellowship in Informatics I have been able to pursue such an ambitious project. This fellowship gave me the freedom to lay the foundations of my research which will now allow important discoveries in neuroscience and developments in machine learning models that will translate to future medical applications.